The tale
It did not take long, before all rooms in the basement of the castle were swamped with huge piles of data chests from kingdoms close to Datamania and empires far far away. The wizards were struggling to keep up with all the chests that the elves brought back to the castle. However, the sheer mass of data chests was not the only source of frustration. It quickly became clear that not all chests had something to do with turning water into gold although both “water” and “gold” were on the label. The wizard Igly shook his head in despair. He was concerned, and they had not even begun to look for chests with the labels “gold” and “H2O”.
– “What’s wrong?” asked Ilo, a small elf with a squeaky voice, gently padding the wizard on the head.
– “Look around you”, sighed Igly. “Look at all these chests. It is good that you bring them here, but many of them are not relevant at all. And I fear that some of the relevant ones are not found”.
– “I see”, replied Ilo, although she didn’t really understand the wizard’s concern. “What’s that sound? Is it… music?”
– “Oh, that sound”, the wizard answered looking almost betrayed. “That is music. Someone came back with a data chest labelled Gold, but it turned out to be music by the lizard Björn Wolfsson”.
– “Sweeeeeet”, Ilo screamed so loud that it could be heard all the way through the castle corridors. “Let’s have some fun and dance for three days and nights. Isn’t this great?”
– “Not really, you see…”, Igly mumbled, but Ilo was long gone
The truth
Humans, and especially machines, can have a hard time interpreting data. Words are ambiguous, and the multitude of spoken and written languages add further to the complexity. Problems range from not being able to interpret the value of a cell due to missing information on the metrics used, to more complex situations where you will have to look for many different terms describing the same object, or stumble across words with different semantic meaning across various disciplines. The same holds for place names and the like.
The FAIR principles address this issue by recommending the use of shared data standards for representing data, and the use of vocabularies and ontologies to represent values and mark-up data. Vocabularies and ontologies are often defined within the research communities and are an unambiguous way of adding semantic meaning to your data. A simple example is to rely on a flower ontology to classify flowers, instead of writing their names in plain text.
Working with data in this way can make your data more useful and discoverable. However, you should be aware that this type of work often has an impact on your methods and the software you use.
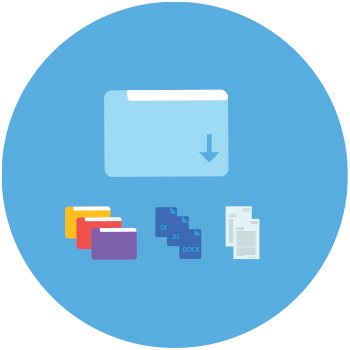